AI/LLM (OpenAI, ChatGPT) Log Management Tools are a specialized category of software designed to streamline and optimize the management of logs generated by artificial intelligence (AI) and large language models (LLMs), such as OpenAI's ChatGPT. These tools are essential for organizations that leverage AI and LLM technologies, as they provide a centralized platform for collecting, analyzing, and monitoring the vast amounts of data produced by these systems.
The primary function of AI/LLM Log Management Tools is to aggregate and organize the logs, making it easier for developers, data scientists, and IT professionals to identify and troubleshoot issues, monitor system performance, and gain insights into the behavior and performance of their AI and LLM applications.
These tools often incorporate advanced data analytics and visualization capabilities, allowing users to explore and interpret the log data more effectively. They may also offer alert and notification features, enabling rapid response to critical events or anomalies within the AI/LLM infrastructure.
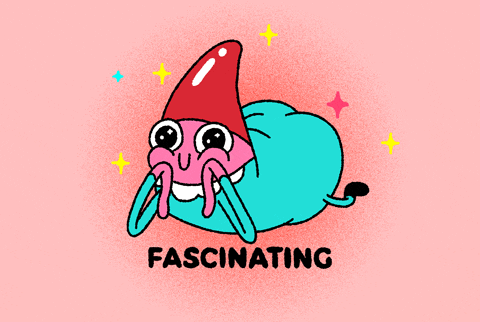
AI Log Management Use Cases
What are the key features and capabilities of AI/LLM log management tools?
How can AI/LLM log management tools improve operational efficiency and decision-making?
What are the key security and compliance considerations when implementing AI/LLM log management tools?
How can organizations evaluate and choose the right AI/LLM log management tool for their needs?
Conclusion
AI Log Management Use Cases
AI Log Management Use Cases
- #1
Automated log file monitoring and analysis to identify anomalies or potential security threats in real-time.
- #2
Utilizing AI-powered tools to aggregate and organize logs from multiple sources for easier access and navigation.
- #3
Implementing predictive analytics to anticipate and prevent system failures or performance issues based on log data patterns.
- #4
Enabling natural language processing to search through logs and extract relevant information for troubleshooting or auditing purposes.
- #5
Integrating machine learning algorithms to auto-categorize log entries and prioritize alerts for more efficient incident response.
What are the key features and capabilities of AI/LLM log management tools?
What are the key features and capabilities of AI/LLM log management tools?
AI/LLM log management tools typically offer a range of features to help organizations effectively monitor, analyze, and manage their logs. Some key capabilities include:
-
Automated log collection and aggregation: These tools can automatically gather logs from various sources, such as servers, applications, and network devices, and consolidate them into a central repository.
-
Real-time log monitoring and alerting: The tools can provide real-time monitoring of log data, allowing users to quickly identify and respond to potential issues or anomalies. They often include customizable alerting and notification systems to ensure timely detection of important events.
-
Advanced log analysis and insights: AI/LLM-powered log management tools leverage machine learning and natural language processing to analyze log data, identify patterns, and generate insights. This can help organizations gain a deeper understanding of their systems, identify root causes of problems, and make more informed decisions.
-
Anomaly detection and predictive capabilities: These tools can use AI and machine learning algorithms to detect anomalies in log data, such as unusual user behavior or security threats, and even predict potential issues before they arise.
-
Compliance and regulatory reporting: Many AI/LLM log management tools offer features to help organizations meet regulatory requirements, such as generating audit-ready reports and facilitating log retention and access control.
How can AI/LLM log management tools improve operational efficiency and decision-making?
How can AI/LLM log management tools improve operational efficiency and decision-making?
AI/LLM log management tools can significantly improve operational efficiency and decision-making in several ways:
-
Enhanced visibility and root cause analysis: By aggregating and analyzing logs from across the organization, these tools provide a comprehensive view of system and application performance. This visibility can help teams quickly identify the root causes of issues, leading to faster problem resolution and reduced downtime.
-
Proactive issue detection and remediation: The advanced analytics and anomaly detection capabilities of AI/LLM log management tools enable organizations to identify potential problems before they escalate. This allows teams to take preventive actions and minimize the impact of incidents.
-
Automated data processing and insights: The AI and machine learning components of these tools can automatically process and analyze vast amounts of log data, generating actionable insights that would be difficult for human analysts to uncover manually. This can lead to more informed and data-driven decision-making.
-
Improved collaboration and knowledge sharing: Many AI/LLM log management tools offer features that facilitate collaboration, such as shared dashboards, workflow automation, and centralized reporting. This can help break down silos and promote a more holistic understanding of the organization's operations.
-
Optimized resource utilization: By providing detailed insights into system and application performance, AI/LLM log management tools can help organizations identify opportunities for optimization, such as load balancing, resource scaling, and infrastructure improvements, leading to more efficient use of IT resources.
What are the key security and compliance considerations when implementing AI/LLM log management tools?
What are the key security and compliance considerations when implementing AI/LLM log management tools?
When implementing AI/LLM log management tools, organizations should consider the following security and compliance aspects:
-
Data privacy and security: These tools handle large volumes of sensitive log data, so organizations must ensure that appropriate data protection measures are in place, such as encryption, access controls, and compliance with data privacy regulations (e.g., GDPR, HIPAA).
-
Regulatory requirements: Many industries have specific regulations and standards governing the collection, retention, and access of log data. AI/LLM log management tools should be configured to meet these requirements, such as providing audit trails, secure log storage, and log retention policies.
-
Model governance and bias mitigation: As AI and machine learning are integral to these tools, organizations must establish robust governance frameworks to ensure model transparency, accountability, and fairness. This includes monitoring for potential biases in the algorithms and their impact on decision-making.
-
Secure integration and access control: AI/LLM log management tools should be securely integrated with other systems and applications, with strong authentication, authorization, and access control measures in place to prevent unauthorized access or tampering.
-
Incident response and forensics: The tools should provide features to support incident response and forensic investigations, such as the ability to quickly retrieve and analyze relevant log data to aid in the investigation and remediation of security incidents.
How can organizations evaluate and choose the right AI/LLM log management tool for their needs?
How can organizations evaluate and choose the right AI/LLM log management tool for their needs?
When evaluating and choosing the right AI/LLM log management tool, organizations should consider the following key factors:
-
Scalability and performance: Assess the tool's ability to handle large volumes of log data and support the organization's current and future growth in terms of data volume, number of sources, and user base.
-
Integration and compatibility: Ensure the tool can seamlessly integrate with the organization's existing IT infrastructure, including various data sources, applications, and security tools.
-
Analytical capabilities and customization: Evaluate the tool's advanced analytics features, such as anomaly detection, root cause analysis, and predictive capabilities. Assess the level of customization and configuration options available to meet the organization's specific requirements.
-
Ease of use and user experience: Consider the tool's user interface, dashboards, and overall user experience to ensure it aligns with the technical expertise and preferences of the IT and operations teams.
-
Security and compliance features: Thoroughly review the tool's security controls, data protection mechanisms, and compliance capabilities to ensure they meet the organization's regulatory and industry-specific requirements.
-
Vendor reputation and support: Assess the vendor's market presence, industry expertise, and the quality of their customer support, as these factors can significantly impact the success of the implementation and ongoing operations.
-
Total cost of ownership: Evaluate the overall costs, including licensing, implementation, training, and ongoing maintenance, to ensure the tool aligns with the organization's budget and long-term financial planning.
Conclusion
Conclusion
AI/LLM Log Management Tools are a crucial component for organizations leveraging artificial intelligence (AI) and large language models (LLMs), such as OpenAI's ChatGPT. These tools provide a centralized platform for aggregating, organizing, and analyzing the vast amounts of log data generated by these advanced technologies.
By offering features like automated log collection, real-time monitoring, anomaly detection, and advanced analytics, these tools help organizations improve operational efficiency, enhance decision-making, and ensure compliance with regulatory requirements. The security and governance aspects of these tools are also critical, as they handle sensitive data and involve the use of AI-powered algorithms.
When evaluating and selecting the right AI/LLM Log Management Tool, organizations should consider factors such as scalability, integration capabilities, analytical features, user experience, security controls, and vendor support, ensuring the chosen solution aligns with their specific needs and long-term strategic objectives.
Similar Categories
Similar Categories